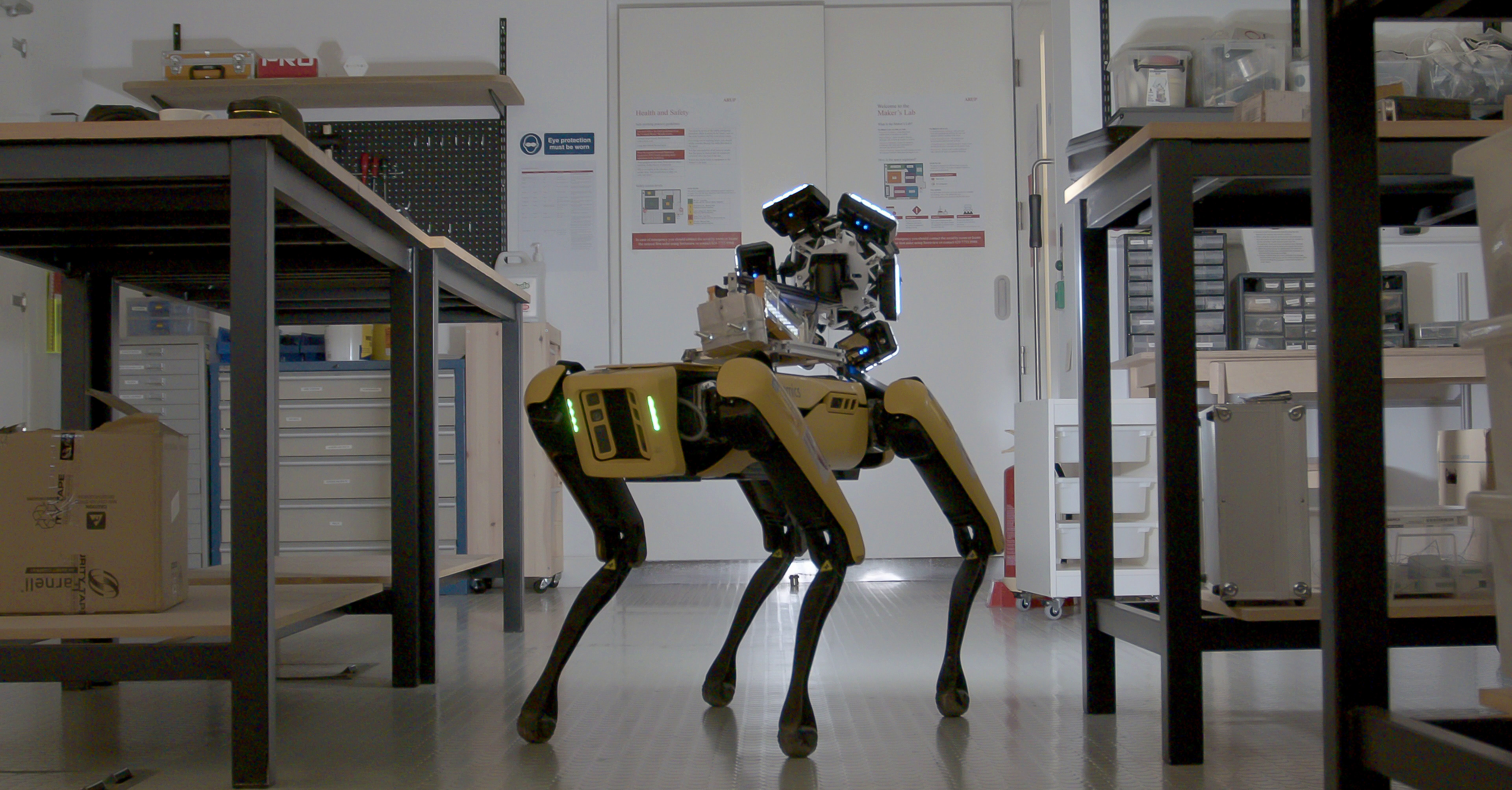
I worked with the infrastructure team at Arup to spearhead an innovative approach to automate and enhance the efficiency of tunnel inspections through the use of 360 imagery, machine learning and robotics.
Tunnel inspections are a time consuming, dangerous, costly and uncomfortable task. By using machine learning and automated capture, it is possible to reduce the risk to human life and greatly improve the reliability and completeness of data capture compared to manual workflows.
You can find a write up of the initial process in this paper.
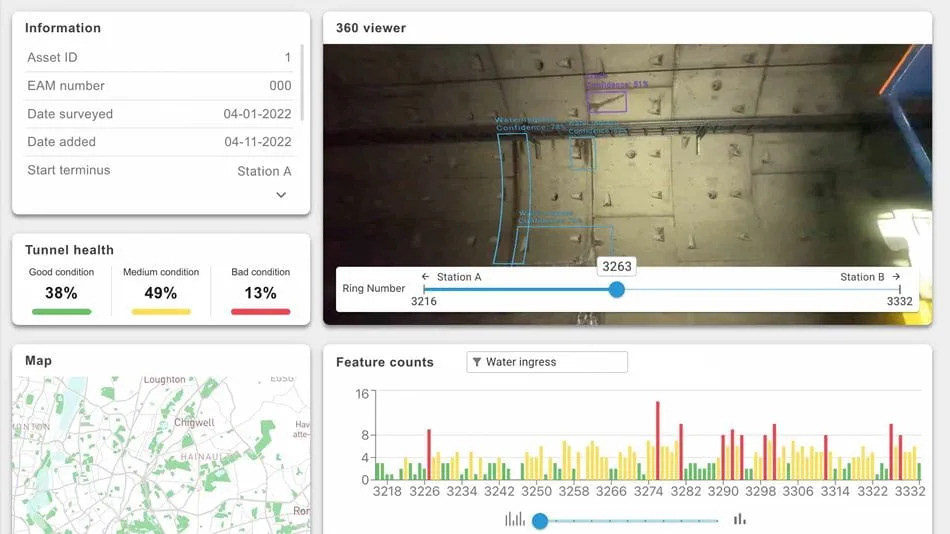
Initial trials were executed by attaching a custom built extrusion frame with a bespoke multi-camera 360 rig with lighting panels to the back of a train. The imagery from the 7 cameras is stitched into an equirectangular image and analysed for defects such as cracks, deposits and water ingress using machine learning systems trained on sample data.
The data is uploaded to Arup's Loupe360 platform where the client can navigate the imagery for each ring with bounding box overlays and view defect reports.
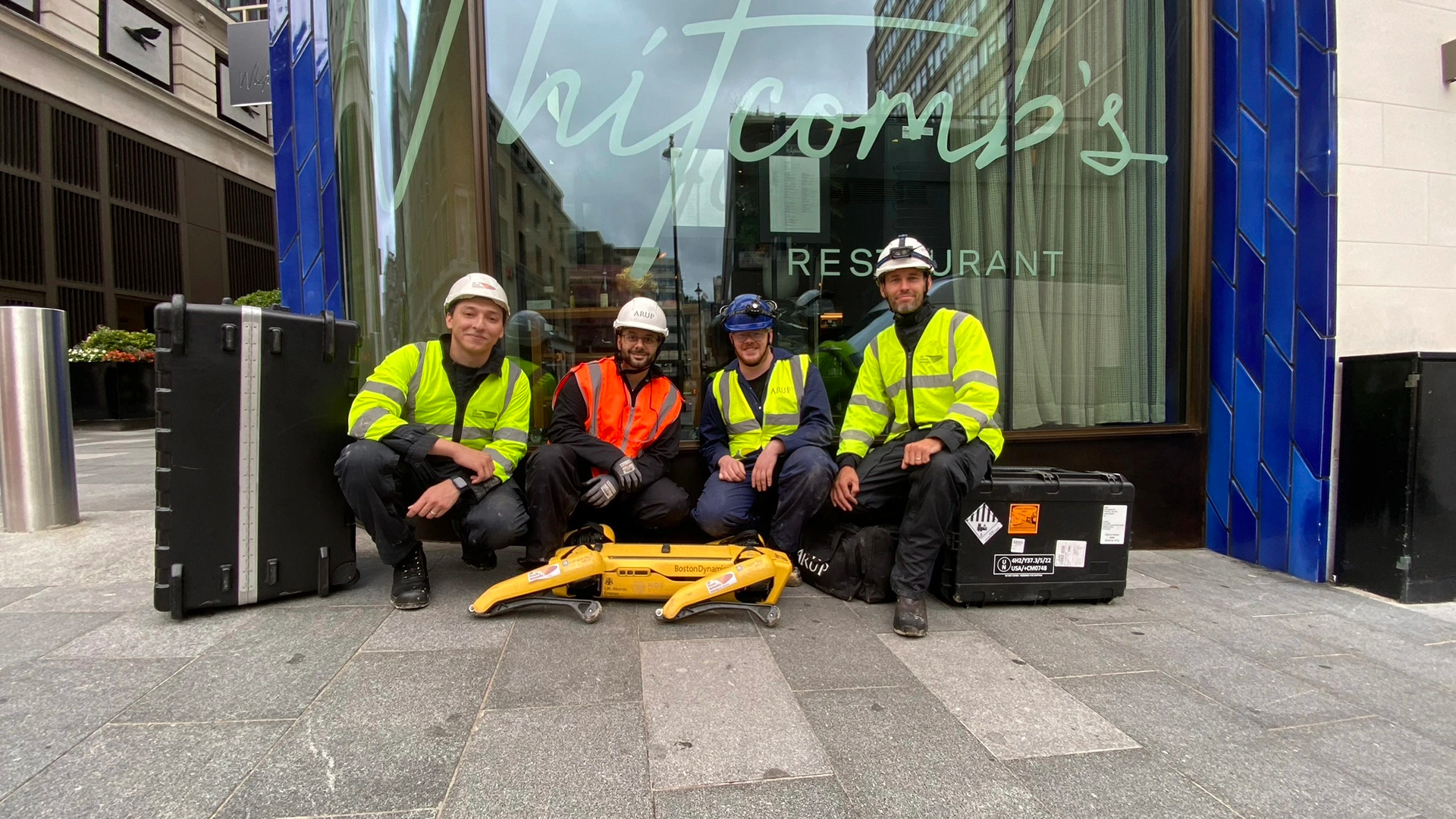
For smaller tunnels, I designed a mounting system for the Boston Dynamics Spot robot including vibration dampeners, mounting points for thermal camera, the 7 camera array and small lighting panels.
To undertake surveys with the robot, I had to complete confined space training which involved crawling through confined spaces while wearing Escape Breathing Apparatus connected to an oxygen cylinder on my back and using a gas monitor. A confined space is defined as enclosed areas with a risk of fire, explosion, loss of consciousness, asphyxiation or drowning. You can see why people might not want to do tasks in these spaces too often!
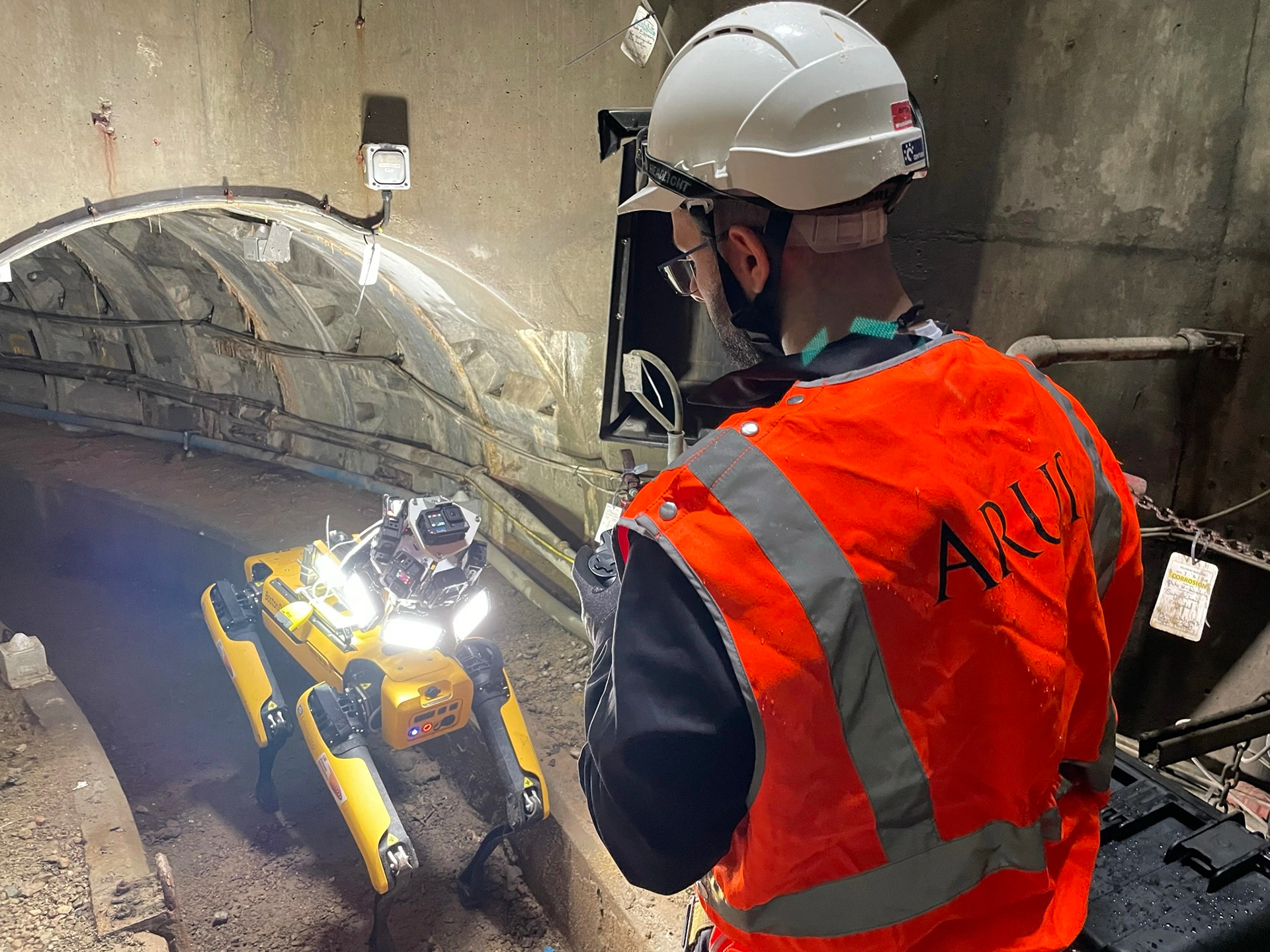